Ideas & advice
Sergey Kondratenko: Big Data and analytics in finance – how to use large volumes of data
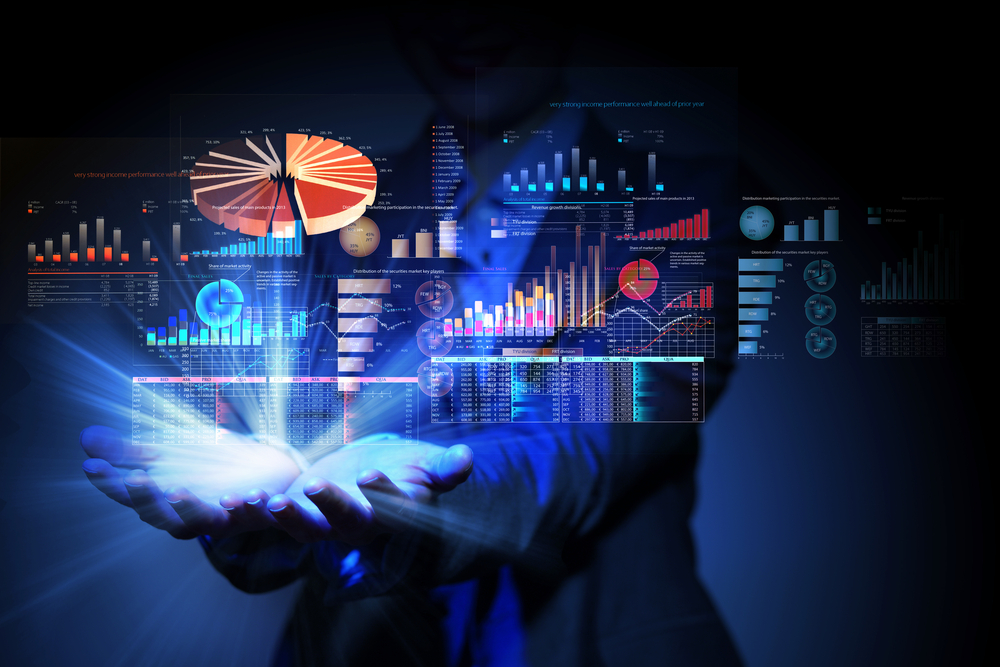
Digitalization of the financial sector has brought new opportunities to it. And this is thanks to technologies such as advanced analytics, machine learning, artificial intelligence, big data processing and cloud computing. Sergey Kondratenko notes that these innovations have significantly changed the competitive environment in the financial sector.
Sergey Kondratenko is a recognized specialist in a wide range of e-commerce services with experience for many years. Now, Sergey is the owner and leader of a group of companies engaged not only in different segments of e-commerce, but also successfully operating in different jurisdictions, represented on all continents of the world. The main goal is to drive new traffic, create and deliver an online experience that will endear users to the brand, and turn visitors into customers while maximizing overall profitability of the online business.
What is Big Data and how is it used in finance?
According to Sergey Kondratenko, Big Data in finance represents huge volumes of data, including structured and unstructured. The specialist adds that this data can be used to predict customer behavior and develop strategies for banks and financial institutions.
The financial industry generates a huge amount of data:
- Structured Data are systematized within the organization to provide key information for decision making.
- Unstructured data in turn, come from various sources in huge volumes and provide significant opportunities for analysis.
A fintech expert reports that billions of dollars worth of transactions are carried out on global financial markets every day. Analysts are responsible for accurately, securely, and quickly monitoring this data to identify patterns and develop predictive strategies. Estimating the value of this data depends heavily on the way it is collected, processed, stored and interpreted. Legacy information systems are not always able to effectively process unstructured and diverse data without significant IT infrastructure costs. In this regard, analysts are increasingly resorting to the use of cloud solutions for processing big data.
– Such solutions not only save costs on local equipment with limited storage capacity, but also increase scalability and flexibility, integrate security into all business processes, and provide effective tools for working with big data and analytics, –says Sergey Kondratenko.
He reports that with the ability to analyze diverse data sets, financial services companies can make informed decisions in a number of areas, such as improving customer service, preventing fraud, optimizing marketing, improving service channel efficiency and assessing risk.
Sergey Kondratenko: How is machine learning used in finance?
As the expert says, machine learning in finance helps analyze large amounts of data and identify important patterns in them. This, in turn, leads to optimization of business processes, making informed decisions and helps forecasting.
– Financial services companies are embracing machine learning as a powerful tool to improve pricing strategies, reduce risk, automate routine operations and gain deeper insight into their customers, behavior Sergey Kondratenko clarifies.
How is machine learning used in fintech? The specialist suggests considering several of the most common examples of the use of this innovative technology.
- Improving client relationships. Financial companies are using machine learning technologies (such as chatbots) to improve the customer experience. In addition, insurance companies often automate the processes of attracting and retaining clients, making them faster and more convenient.
- Attracting Customers and the Internet of Things (IoT). Attracting customers is another important area of application of machine learning and artificial intelligence, emphasizes Sergey Kondratenko. IoT devices generate large amounts of data that help understand customer behavior and preferences. This data, according to the expert, can be used to create personalized marketing campaigns or improve customer service methods. Improving the customer experience gives them a reason to trust that company rather than leaving for competitors.
- Security analysis and portfolio management (robo-advisors). Robo-advisors provide an excellent example of the application of machine learning in the financial industry. These online services help users create and manage their investment portfolios. They adapt to clients’ preferences and use this information to make decisions about how best to manage investments.
- Fraud Detection. Sergey Kondratenko points out that machine learning models learn to identify patterns based on data. This ability helps identify normal behavior and detect suspicious activities such as money laundering or insider trading.
- Risk management and prevention. Machine learning technologies help identify risks based on historical data and probability statistics. Machine learning can also be used to assess possible scenarios and develop risk management strategies.
- Analysis of unstructured and big data. Machine learning in finance makes it easier to analyze unstructured data such as contracts or financial statements.
- Big Data Analysis to Gain Competitive Advantage.
– Big data analysis is becoming increasingly important for understanding customer behavior and trends, says Sergey Kondratenko. – Machine learning and artificial intelligence help process huge amounts of data, identify patterns and make predictions. This can give companies a competitive advantage, helping them make better decisions faster than their competitors.
- Loan underwriting. The use of machine learning algorithms makes it possible to analyze large volumes of data to create more accurate and efficient loan underwriting models. This helps fintech companies reduce time and costs for loan applications and improve the efficiency of lending decisions.
Machine learning also plays an important role in data protection and privacy. The main aspects and problems associated with this are listed by Sergey Kondratenko:
- Confidentiality of customer data. Financial services companies handle sensitive customer data, such as accounts and personal data, and must ensure it is protected from leaks and unauthorized access.
- Data privacy. To prevent customer identification, it is important to anonymize data before using it in machine learning models.
- Learning from customer data. Fintech companies use customer data to train models, but must be transparent and obtain customer consent to use their data.
- Legislation and regulation. Different countries have different legal regulations on data protection, and financial companies are required to comply with them.
- Security of machine learning models. Machine learning models can be subject to attacks, and their security requires constant monitoring and protection.
- Privacy-aware training. Machine learning methods are being developed that allow learning from data without revealing its content, which helps maintain its confidentiality.
- Documentation and reporting. Financial services companies must comply with documentation and reporting requirements regarding the use of data and machine learning models.
In conclusion, Sergey Kondratenko notes that machine learning has a significant impact on the fintech industry. Fintech companies can use machine learning to create innovative products and services, increase customer trust and manage risks more effectively.
In turn, the use of big data and analytics in finance has enormous promise and can transform this industry. Here are a few key areas where this technology can be effectively used: market forecasting, risk analysis, credit scoring, anti-fraud, personalized service, customer service. Additionally, big data analytics can help financial institutions develop more accurate and strategic plans for the future, taking into account various economic and market factors. In general, the use of Big Data, analytics and machine learning opens up new horizons for financial companies. Enables them to operate more efficiently, provide quality services and make informed decisions in a dynamic financial environment.
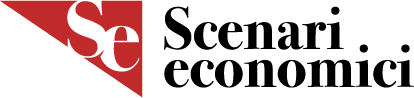